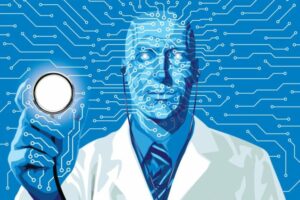
What is artificial intelligence?
Artificial Intelligence (AI) is the ability of a computer to perform tasks commonly associated with intelligent beings. The goal of AI is to produce a machine whose intellectual ability is indistinct from that of a human being. Machine learning is a branch of AI that focuses more specifically on the use of algorithms to produce programs that mimic the way that humans learn, to improve its overall accuracy. Over the past decade, the rise of AI has continued and we have started to see it begin to spread its impact across various fields.
With this project, I explore the use of AI in the medical field and more specifically examine how it is already being used throughout the United States. Black-box medicine is a term that I came across vastly in my research and this refers to the idea that decisions made by AI are not yet understandable because the system itself is not yet understood. It is for this reason that many physicians are not yet willing to adopt AI in their own practice of medicine. With further research into the algorithms that exist behind AI systems, it is likely that more physicians will be willing to support its use in the near future.
Why could this useful be useful in medicine?
Machine learning algorithms can quickly process large amounts of clinical documentation, identify patterns in the data and make predictions about medical outcomes. This could be incredibly useful for the future of medicine and could change the medical field as we know it.
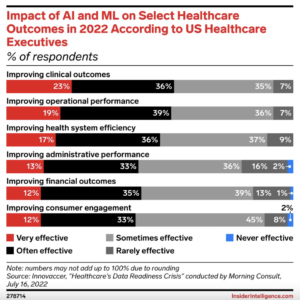
ChatGPT, which is an AI chatbot that is currently being used by millions around the world, has already proven itself in the realm of medicine. Research has looked at the abilities of chatGPT concerning complicated medical knowledge in which physicians spend hours throughout their medical education learning, and have already found it to be incredibly reliable. According to Dr. Victor Tseng of Ansible Health, the program has shown the ability to pass the medical licensing exam. It was not only able to diagnose pretend patients in hypothetical scenarios, but it was able to answer questions within 5 seconds and with 60% accuracy. While this isn’t perfect, this is impressive considering finding these answers to the exam questions on Google or with an open-book approach would probably take multiple hours. It is due to these abilities that some people believe there is huge potential for this type of technology within the medical field.
Another way that AI could be incredibly beneficial to hospitals and even smaller practices, is its ability to serve as administrative assistants. Keeping track of documents and individual patient information can be very time-consuming, but quickly be solved with something like AI. With machine-based learning, programs can be created to supplement the work of administrative roles.
Radiology has been one of the most promising areas of AI influence in the medical field, with the ability of AI software to read and interpret X-rays. These algorithms are developed by teaching systems what to look out for in images based on previous patient examples. For instance, presenting AI with various images of the same fractured body part can teach it to distinguish a broken bone from perhaps just a bruised one. Despite these abilities, it is important to recognize that supporters of AI in medicine are not encouraging the replacement of physicians but rather using it as a supplement to improve human abilities. In the case of a radiologist, AI would be used in addition to their own X-ray readings to confirm their findings and perhaps draw their attention towards things they would otherwise miss.
Below is an interactive map to explore some places throughout the United States that have already started to explore the use of AI in medicine (Data retrieved from sources that can be found in my bibliography).
AI already has shown success.
Dr. Jakob Weiss of Massachusetts General Hospital led some promising research on the use of AI to determine a patient’s risks for developing cardiovascular problems. Chest X-rays are the most common type of radiological exam which is why they are being prominently used for AI research. He and his colleagues found there to be information captured by the chest X-ray that they were not aware of and had no way to quantify, however using deep learning techniques they were able to create a program to extract this information and use it for prognosis. The algorithm was trained using 150,000 X-rays from almost 41,000 patients, as well as patient history and outcome. It was then further tested on a series of additional X-rays but without any additional patient information, and using the images alone it was able to determine the risks of these patients. If approved by the FDA, this type of AI technology could be used to add value to patient exams.
Further research has looked at the role of AI as an early-screening diagnostic tool for cancer. In one case, AI was able to pick up on a patient’s breast cancer four years before her actual diagnosis. It can look at mammograms and identify areas of the image that radiologists should look at more closely. Rather than actually diagnosing cancer in its earliest stages, AI instead provides the information needed to tell patients that they are at high risk for developing cancer before they actually develop it. This means that patients and their physicians are more cautious in examining for cancer to ensure it is not overlooked and is diagnosed as soon as possible. Once again, this study emphasized that this technology exists to help human decision-makers as opposed to outright replacing medical professionals. According to the New York Times, this use of AI technology in the screening of breast cancer has reduced the workload of radiologists by 30 percent and has increased cancer detection by about 13 percent.
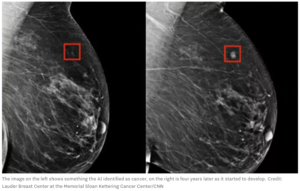
Existing Problems with AI
While AI has been extremely promising, particularly in the domain of radiology, there are still multiple problems with it that need to be addressed before it can become more widely used in the medical field.
One of the first examples I came across while looking at current uses of AI in medicine was a case at the University of Michigan where AI was being used to alert physicians to possible cases of sepsis in their patients. After investigating its performance, the researchers realized that it was performing worse than they had anticipated, flagging cases of sepsis that did not exist and missing actual cases of the condition. It is cases like this that make physicians skeptical about using AI in their own practice of medicine. However, these failed cases must exist, so that researchers can work on developing more accurate algorithms. This specific article I came across, emphasizes the importance of validating the system through its manufacturing stages to avoid later complications. These systems constantly need to be updated with new information and go through troubleshooting for potential errors.
Another case in which AI demonstrated shortcomings within the medical field was in its use to diagnose covid patients. At the height of the pandemic when physicians were desperate for new ways to quickly diagnose COVID-19 patients, they turned to AI. Many hospitals were releasing data on their covid cases including patient radiographs. Using this data, as well as lung X-rays of healthy patients collected by the US National Institutes of Health, an algorithm was formed to diagnose patients. However, as they looked deeper into AI technology they became aware of limitations in the algorithmic design. For instance, the technology was picking up on little differences in X-rays that were solely due to different radiological practices across hospitals. The letter “R” or “L” to label a person’s right or left side was being included in the algorithm so that the system was diagnosing patients based on the style and placement of the letter as opposed to providing an actual medical diagnosis.
Text Analysis
Below are two text analyses I completed for articles and papers I have used throughout this project. I grouped certain sources based on whether I classified them as in support or against AI in medicine. As you can see from the word clouds I made below using Voyant, the first displays negative words like “limitations” and “threatening”, while the second displays more positive words like “opportunities” and “potential”. Based on my research, I find that these two visuals successfully capture the ongoing debate and opinions surrounding the use of AI in medicine.
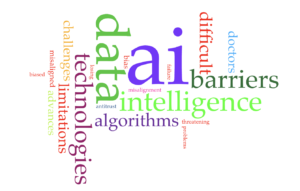
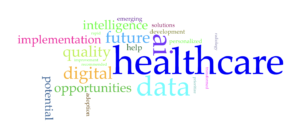
Physician Concerns
Many physicians are concerned about what AI could mean for their own careers in medicine. With a new reliance on machine-learning algorithms in the medical field, many physicians are worried they would experience a sense of detachment from their work. Currently, they have a decent amount of authority over their patients and how they are treated, but the concern is that they would lose this in the presence of AI. Additionally, physicians anticipate that AI will dissolve the patient-provider relationship which is typically quite strong. Physicians are more than just providers, developing strong connections with their patients that creates an atmosphere of trust. Despite the numerous AI studies that have discussed how the technology would be used as an additional tool, rather than a replacement for medical professionals, physicians are still threatened by job security. based on what I have read, I don’t see AI taking over to such an extent that physicians will no longer be useful, however, I do understand these concerns. Although these are all valid concerns, the lack of understanding about how these algorithms work seems to be the most worrying among physicians. There is still vast research that needs to be conducted before physicians are going to be able to develop the trust needed to feel comfortable in adopting this new technology.
AI Bias
AI produces software that can personalize and improve medicine greatly, however in doing so they are creating bias. There have been previous studies where AI has made decisions about medical treatment that are often disadvantaged to communities of color. These tools are often built through the use of homogenous data sets that are not reflective of the population at large, so it is not surprising that there is bias. For example, black communities are four times more likely to develop kidney failure, however an algorithm that was created to determine placement on the transplant list, puts black patients lower than white patients. It is for reasons like this that there is a lack of trust among physicians to use AI. No physician should have to worry that bias within an algorithm could prevent their patient from receiving the care they deserve and need.
How can AI gain the trust of physicians?
Looking ahead, I do believe that there will be a place for AI in the medical field, and think it could provide incredible, even life-saving benefits. However, I think the only way for it to be successful is for it to have 100 percent approval from physicians. Going forward, more research needs to be conducted to ensure the best possible technology and as discussed previously, this technology must be continuously upgraded based on new data to ensure accuracy and the best possible care for patients. Although this project focuses on the physicians’ relationship with AI, I think the patients’ relationship with it is just as important and will only be able to develop in the presence of physician approval.
References
Adler-Milstein, J., Aggarwal, N., Ahmed, M., Castner, J., Evans, B. J., Gonzalez, A. A., James, C. A., Lin, S., Mandl, K. D., Matheny, M. E., Sendak, M. P., Shachar, C., & Williams, A. (2022). Meeting the Moment: Addressing Barriers and Facilitating Clinical Adoption of Artificial Intelligence in Medical Diagnosis. NAM perspectives, 2022, 10.31478/202209c. https://doi.org/10.31478/202209c
Christensen, D., Manley, J., Resendez, J. (September 2021). Medical Algorithms Are Failing Communities of Color. Health Affairs. https://www.healthaffairs.org/do/10.1377/forefront.20210903.976632/
Christensen, Jen. (2023, February 3). Paging Dr. AI? What ChatGPT and artificial intelligence could mean for the future of medicine. CNN. https://www.cnn.com/2023/02/02/health/artificial-intelligence-medicine/index.html
Fornell, Dave. (2023, January 12). AI predicts heart disease risk using single chest X-ray. Radiology Business. https://radiologybusiness.com/topics/artificial-intelligence/video-ai-predicts-heart-disease-risk-using-single-chest-x-ray
McCall, Jonathan. (2023). Building Better Guardrails for Algorithmic Medicine. Duke AI Health. https://aihealth.duke.edu/building-better-guardrails-for-algorithmic-medicine/
Poon, A. I. F., & Sung, J. J. Y. (2021). Opening the black box of AI-Medicine. Journal of gastroenterology and hepatology, 36(3), 581–584. https://doi.org/10.1111/jgh.15384
Quinn, T. P., Jacobs, S., Senadeera, M., Le, V., & Coghlan, S. (2022). The three ghosts of medical AI: Can the black-box present deliver?. Artificial intelligence in medicine, 124, 102158. https://doi.org/10.1016/j.artmed.2021.102158
Savage, Neil. (2022, March 29). Breaking into the black box of artificial intelligence. Nature. https://www.nature.com/articles/d41586-022-00858-1